Table Of Contents
What Is Spatial Regression?
Spatial regression refers to a statistical technique utilized to evaluate the association between independent and dependent variables considering data's spatial dependence. It aims at exposing spatial patterns, examining variable correlations, taking geographical locations into account, and forecasting for particular areas.
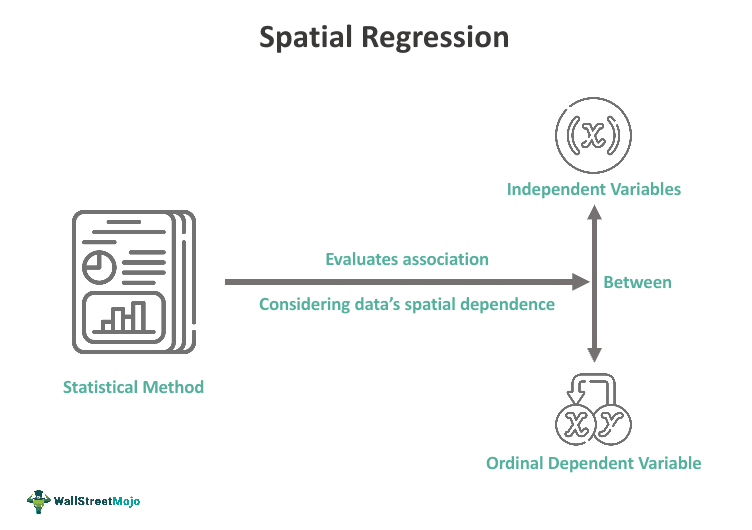
It applies to geographically referenced data like crime rates, disease prevalence, and housing prices. Moreover, it finds usage in miscellaneous fields like economics, urban planning, environmental science, plus public health. Moreover, spatial regression models help address issues such as spatial autocorrelation, spatial heterogeneity, and spatially clustered observations.
Key Takeaways
- Spatial regression is a statistical method employed to assess the connection between independent and dependent variables. At the same time, these take into consideration the spatial relationships within the data.
- Its primary objectives include revealing spatial patterns, scrutinizing relationships between variables, incorporating geographic locations, and making predictions for specific areas.
- Moreover, its significant assumptions of regression include spatial autocorrelation, linearity, homoscedasticity, independence of errors, and no endogeneity.
- Hence, spatial regression finds application in diverse fields, including economics, geography, environmental modeling, public health research, crime analysis, urban planning, and peer-to-peer credit risk management.
Spatial Regression Analysis Explained
Spatial regression is a statistical method modeling relationships amongst variables while accounting for their geographical context. It introduces geographical or space context into the regression framework. Hence, it predicts the exact data's current location and forecasts future locations spread across other cities. Moreover, this model integrates spatial information into traditional regression models through a spatial weight matrix.
Such a matrix has weights depicting spatial relationships amongst all observational pairs per specific criteria like contiguity or distance. After creating the weight matrix, the regression model is modified by incorporating the spatial lag term into the model. Therefore, the spatial weights matrix defines the proximity or influence between observations. Commonly used weightings include binary weights or distance-based weights.
Moreover, the spatial lag term itself is the mean of the dependent variables between neighboring observations. Thus, this lag term also accounts for spatial autocorrection. Furthermore, the lag term in regression can introduce spatial dependence into the observations.
Talking of its implications, it has many, like allowing identification and quantification of the spatial patterns of variables, helping to recognize regions with high or low values, and comprehending the distribution of the variable. This model considers the physical position of the variables. Therefore, it examines the correlations between them, like how crime rates in one neighborhood affect another.
Since it has versatility, it gets applied to different data types like areal data, network data, and point data. Additionally, it addresses dynamic research questions, studies relationships and spatial patterns between variables and forecasting, plus evaluates the policy impacts. In addition, these models provide a more accurate representation of the spatial structure in the data. Thus, it can lead to better-informed policy decisions and predictions.
Assumptions
Spatial regression analysis uses many assumptions to evaluate the relations between more than one independent and a dependent variable. Below, we list some of the significant assumptions.
- Spatial autocorrelation: Closer observations show more similarity, which is an essential premise that permits modeling spatial dependency in regression.
- Linearity: In order for it to work, dependent and independent variables must have a linear connection that holds at all variable levels.
- Homoscedasticity: To obtain unbiased coefficient estimations, we assumed that the error term variance remains constant across values of the independent variable.
- Independence of errors: For unbiased coefficient calculations. It considers the error term variance to be constant across values of the independent variable.
- No endogeneity: To avoid biases resulting from missing data or measurement mistakes. This model assumes no association between error terms and independent variables.
Examples
Let us take the help of a few examples to understand the topic.
Example #1
The 2019 research paper "A Spatial Regression Analysis on Neighborhood-Level Trust and Cooperative Behaviors" explores the use of spatial regression to analyze social influence. Instead of administrative boundaries, it introduces a fresh method of defining communities based on physical distances. It also includes previous studies linked "neighborhood-level trust" with residents' well-being. In the UK, the study, using 250 postcode regions, identified a positive connection between trust and good self-rated health. In Brisbane, Australia, where the city is divided into 82 statistical local regions, high collective efficacy significantly reduced violent victimization rates.
On a global scale, the study found a negative correlation between individual-level robbery victimization and national-level generalized trust. Trust in the community fosters cooperation, benefiting crime prevention and health. Hence, the study acknowledges that different neighborhood sizes can affect results, addressing this issue through a spatial approach that considers physical proximity.
In summary, this research introduces an innovative approach to spatial neighborhood analysis, emphasizing the importance of trust and proximity. Further research needs validation of this technique, especially in terms of using comprehensive psychological assessments. The small sample size may have influenced the findings of the multilevel models.
Example #2
Let's consider a practical example of spatial regression in the context of real estate prices. Suppose Annie is interested in understanding the factors that influence housing prices across different neighborhoods in a city. Hence, she collects data on housing prices (the dependent variable), as well as various independent variables such as the size of the house, proximity to public transportation, and neighborhood crime rates. Since she suspected that the prices might influence housing prices in one neighborhood in nearby neighborhoods, she decided to apply a spatial regression model.
In this spatial regression analysis, she constructed a spatial weights matrix to represent the spatial relationships between neighborhoods. After specifying the model, which includes both traditional predictors (like house size and crime rates) and a spatial lag term, she estimated the coefficients using spatial regression techniques.
Therefore, the results of the model could reveal exciting insights. For instance, she might find that the spatial lag term, representing the average housing prices in neighboring areas, has a significant impact on the housing prices in a given neighborhood. Thus, this suggests the existence of spatial autocorrelation, indicating that the prices indeed influence the prices of houses in one area in nearby areas.
Applications
Spatial regression widely applies and finds use in various domains. We list some of its applications below.
- Spatial Econometrics: In economics, it plays a crucial role in modeling geographically dependent data to comprehend the impact of location on economic factors such as house prices and regional development.
- Geographical Analysis: It helps in geography to understand how location affects phenomena like population distribution, land use, transportation networks, and environmental processes by revealing correlations between geographic variables.
- Environmental Modeling: In order to forecast ecological changes, environmental science uses it to examine how environmental factors—such as temperature and precipitation—interact with ecosystems.
- Public Health Research: Epidemiologists use spatial regression as a tool to analyze disease spatial patterns and the factors influencing them. This helps with risk assessment and intervention targeting.
- Crime Analysis: In criminology, it provides geographical patterns of crime as well as local features and socioeconomic situations that affect crime rates. This information aids in the development of efficient crime prevention tactics.
- Urban Planning: It determines which locations have high or low crime rates, forecasts how new projects will affect traffic, and evaluates how zoning laws will affect the cost of homes.
- Public Health: It analyzes the transmission of infectious diseases, pinpoints regions with high prevalence of chronic illnesses, and assesses the success of public health initiatives.
- Environmental Science: It examines the distribution of pollutants, projects the potential impact of climate change on ecosystems, and assesses the efficacy of environmental regulations.
- Economics: It examines the distribution of economic activity, forecasts how policies will affect local economies, and evaluates the contribution of transportation infrastructure to economic growth.
- Peer-to-Peer Credit Risk Management: it improves financial risk management By assisting banks with credit risk assessment and credit risk contagion impact measurement.
Spatial regression analysis, including the use of spatial regression models and spatial regression in R, has found diverse applications in various fields. Additionally, spatial regression discontinuity designs have been utilized to evaluate the effectiveness of policy interventions, such as examining the effects of educational programs on student performance based on geographical boundaries.