Table Of Contents
What Are Instrumental Variables?
An Instrumental Variable (IV) refers to a third variable that gauges the impact of the exogenous regressor associated with the explanatory variable on the response variable, which has a negative correlation with such regressor. Thus, it helps depict the unexpected behavior of a regression model.
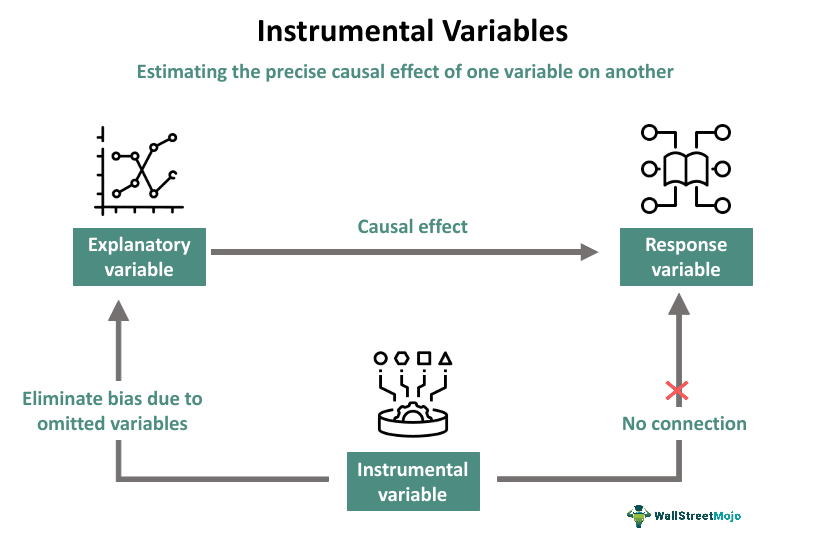
The instrumental variable estimation is a widely used method in econometrics, health sciences, social sciences, finance, and epidemiology for analyzing the actual correlations between dependent and independent variables. It is effective since it serves as a fix to the incompetence of addressing the endogeneity of the predictor variable, which is a crucial factor in regression analysis.
Key Takeaways
- An instrumental variable or instrument denotes a third variable (Z) that addresses the endogenous variables influenced by other factors in a regression analysis model.
- It aims to gauge the unexpected interaction behavior in a regression model, revealing the true correlation between the explanatory variable (X) and the response variable (Y).
- The most important instrumental variable conditions to consider are relevance, exogeneity, exclusive restriction, and exchangeability.
- The selection of IVs involves adopting a comprehensive approach, combining theoretical knowledge, considering exogeneity and correlation criteria, using multiple data sources.
Instrumental Variables Explained
The instrumental variable regression is a commonly employed statistical tool in econometrics, social science, health science, and epidemiology. It divides the explanatory variable into two parts where one shows a correlation with the error term ε and the other is not correlated to ε. This methodology allows the estimation of the regression equation while controlling for confounding variables, simultaneity, omitted variable bias, measurement errors, and reverse causality.
IV is correlated with the explanatory variable, but it does not affect the response variable directly. It only estimates, corrects, and highlights the effectiveness of the causal link between the explanatory and response variables.
The challenge with ordinary least squares (OLS) lies in its susceptibility to inconsistency due to the endogeneity of the independent variable X, where changes in X are not only linked to changes in Y but also to changes in the error term ε. An ideal solution involves generating exogenous variation in X.
Although experiments offer a reliable approach, they are often expensive and infeasible in many economic applications. Thus, researchers need to explore alternatives like the IV method for deriving genuine outcomes by considering the endogeneity factor.
Despite its utility, instrumental variable regression lacks universality as it may not always be feasible for all types of exogenous regressor analysis. Moreover, various methods and tools utilizing available data can be employed to strengthen or challenge IV assumptions and estimate the extent or direction of possible bias if the conditions are not met perfectly.
In finance, instrumental variables can be used to evaluate the effects of monetary policy changes on various sections of the society. Aspects like public expenditure, household income, and investment readiness can be measured or estimated based on the parameters undertaken for study. As part of the corporate finance function, IVs can help executives understand the causal relationships between various key financial health indicators like capital expenditure, budgeting, working capital management, etc.
Assumptions
The instrumental variable method is contingent upon the following essential conditions for its validity since failure to meet the same can result in biased or inconsistent estimates:
- Relevance: The instrumental variable must exhibit a correlation with the endogenous explanatory variable, ensuring a substantial impact on the independent variable of interest.
- Exogeneity: The IV should remain uncorrelated with the error term in the regression equation, preventing biases and ensuring that it does not directly influence the dependent variable through channels other than the endogenous variable.
- Exclusion Restriction: Such a variable should solely influence the dependent variable while indirectly impacting the outcome. Hence, it should not be correlated or directly affect the dependent variable.
- Exchangeability: The effect of IV on the outcome must be unconfounded. It means there should not be any other variables simultaneously affecting both the IV and the outcome. If such variables exist, they will likely skew the results.
While these conditions are fundamental for IV analysis, additional criteria may be necessary to identify causal effects. For instance, just like the exchangeability assumption, in traditional epidemiologic approaches, IV analysis relies on unverifiable conditions. However, the reasonability of these assumptions is still debatable on specific grounds, affecting the reliability of outcomes in some cases.
When To Use?
Although traditionally prevalent in economics research, instrumental variables are increasingly finding application in epidemiological studies. Today, IVs serve as a critical tool in various disciplines, including statistics, econometrics, epidemiology, and related fields.
Instrumental variables (IV) methodology finds application in estimating causal relationships, especially when controlled experiments are impractical or when every unit in a randomized experiment cannot receive treatment or assignment successfully.
Comparable to propensity scores, IVs can account for both observed and unobserved confounding effects. While other methods like stratification, matching, and multiple regression can only address observed confounders, IVs provide a unique approach. Moreover, in the regression discontinuity model, instrumental variable methods facilitate the identification of the Complier Average Causal Effect (CACE) with the instrument's eligibility for threshold. CACE shows compliers by choice and those by chance (or without intending to) when analyzed in a specific situation.
How To Choose?
It is crucial to opt for proper instruments that exhibit a correlation with endogenous variables while maintaining independence with reference to the outcome. Another critical factor is these instruments meet the relevance condition by having a strong correlation with the endogenous variable. However, identifying suitable instrumental variables poses a challenge and necessitates an understanding of the model's structure and theory.
Some fundamental criteria are stated below, where X is the independent variable, Y is the dependent variable, and Z is the selected exogenous variable.
- Exogeneity (Cov(Z,ε) = 0): The selected variable Z must be exogenous, indicating that other variables do not influence it in the system. Although direct testing is impractical, reliance on theoretical knowledge, particularly within economic theory, is essential for determining exogeneity.
- Correlation with Endogenous Variable (Cov(Z, X) ≠0): Z should demonstrate a significant correlation with the endogenous explanatory variable X. A robust first stage, characterized by a substantial correlation, is pivotal for the effectiveness of IVs. Weak correlations may result in unreliable estimates for parameters and standard errors.
IVs cannot be identified through direct regression with the actual data. Instead, it necessitates the reliance on the understanding of the model's structure and the theoretical foundations of the experiment. If feasible, it is advisable to incorporate two different data sources for instrumental variables. This approach enhances the robustness of the analysis and ensures the independence and relevance of the chosen instruments.
Collecting longitudinal data proves valuable in identifying such variables. Also, if a mediating variable is known to influence both the independent variable (X) and the dependent variable (Y), it can serve as a potential instrumental variable. This is particularly beneficial when considering causal pathways and mediating effects.
Examples
There have been various experiments in the real world where instrumental variables are used to identify the reasons behind the different behavioral patterns of a regression model. Let us discuss some relevant examples in this section.
Example #1
Suppose an economist experiments on the indirect impact of corruption on poverty in a developing nation. In his research, he finds that corruption significantly impacts the distribution of government resources designated for poverty alleviation programs. In this scenario, the instrumental variable could be the level of corruption within the government, gauged or tested by metrics such as embezzlement or bribery within public service.
As corruption intensifies, funds allocated for poverty reduction may be misappropriated, leading to an unequal distribution of resources. The instrumental variable, representing the influence of corruption on inequality, plays a crucial role in diminishing the efficacy of poverty alleviation initiatives.
The escalation of corruption results in a skewed allocation of resources, exacerbating income disparities. This, in turn, contributes to a heightened prevalence of poverty, as resources meant for the most vulnerable segments of the population are redirected due to corrupt practices in resource distribution.
Example #2
Signifying the instrumental variables in econometrics, let us take another example where researchers aim to explore the factors influencing medical expenses, incorporating the endogenous regressor of having health insurance and exogenous regressors, including illnesses, age, and income.
The chosen instrumental variables for this investigation are the Social Security (SS) income ratio and the existence of the firm in multiple locations. The dataset used for the analysis is sourced from the Medical Expenditure Panel Survey (MEPS), a US government department that publishes statistics and data about medical expenditure patterns in the US.
In the OLS model, the coefficient for health insurance suggests medical expenses are 7.5% higher for those with insurance. However, in the two-stage least squares (2SLS) model, after instrumentation, the coefficient changes significantly, indicating an 85.2% reduction in medical expenses for individuals with health insurance compared to those without. Notably, the 2SLS coefficient differs from the OLS coefficient, highlighting the impact of endogeneity in the analysis.
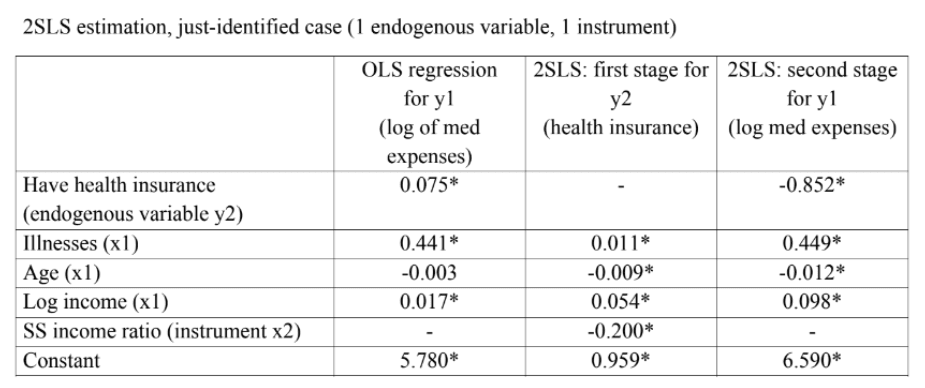
Example #3
A research-driven study from May 2016 talks about the causal relationship between income and health, highlighting how financial credits might affect health outcomes. Researchers aimed to analyze whether financial credits impact factors like health, even when they are not considered direct income in an individual’s hands.
In this case, the credits individuals receive become an instrumental variable for studying the causal inferences arrived at due to the interaction between income and health.