Table Of Contents
What Is Spatial Econometrics?
Spatial Econometrics is a subfield of economics that deals with analyzing economic data in the context of space or geography. This field has gained importance recently as researchers and policymakers seek to better understand regional and urban economic issues.
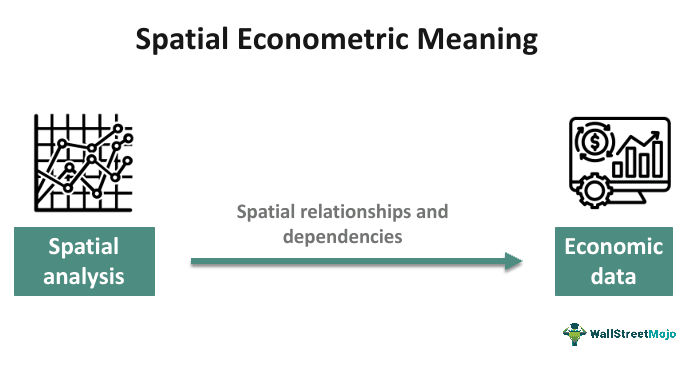
It is primarily concerned with the impact of spatial relationships and spatial dependencies on economic phenomena. It is a specialized branch of economics that deals with the formal specification, testing, and estimation of empirical models while considering the presence of spatial externalities. These spatial externalities can manifest in the form of spatial interactions or spatial spillover effects.
Key Takeaways
- Spatial econometric models are statistical models used to analyze and account for spatial dependencies or relationships in data.
- It captures the spatial autocorrelation, spatial heterogeneity, and spatial externalities that may be present in the data.
- The goal of spatial econometric models is to provide a more accurate and realistic representation of the data, considering the spatial context.
- It helps researchers and policymakers better understand the spatial interdependence of observations and its implications for decision-making and policy formulation in various spatially related domains such as real estate, health and agriculture.
Spatial Econometrics Explained
Spatial econometrics is a specialized field within economics that focuses on analyzing and modeling economic data in a way that considers the inherent spatial relationships and dependencies among observations, which can be regions, points, or areas in close geographical proximity.
In a regression context, spatial effects encompass two primary categories of specifications: spatial dependence and spatial heterogeneity. These concepts are essential in spatial econometrics and play a crucial role in understanding how spatial relationships impact regression models.
Spatial dependence acknowledges the spatial interdependence of observations in different locations, while spatial heterogeneity recognizes variations in relationships or error structures across locations. Thus, spatial econometric models address these effects, enabling researchers to build more accurate and insightful models for analyzing data with spatial dimensions.
The spatial econometric model is an extension of the linear regression model (Y=Xβ+ε) that incorporates various forms of spatial effects. This model accounts for both endogenous and exogenous spatial interactions, as well as interaction effects among the error terms.
Let us break down the components and their meanings:
#1 - Dependent Variable (Y)
Y represents a vector of the dependent variable for each unit in the sample (i = 1, ..., N). In other words, it contains observations of the variable that one tries to model for each unit or location in our data set.
#2 - Independent Variables (X)
X is an N×K matrix of exogenous explanatory variables. These are the variables that we believe influence the dependent variable. Here, each row in the matrix corresponds to a unit or location, and each column represents a different explanatory variable.
#3 - Endogenous Interaction Effect (ρWY)
ρ represents a parameter that captures the strength of endogenous spatial interaction effects.
W is an N×N nonnegative matrix that describes the spatial arrangement of the units in our sample. It defines how units are related to one another in space.
The term ρWY represents the endogenous spatial interaction effect, which signifies the impact of the dependent variable (Y) of one unit (e.g., unit A) on the dependent variable of another unit (e.g., unit B). Thus, it accounts for the spatial interdependence between units.
#4 - Exogenous Interaction Effects (WXρ)
ρ is a vector of parameters associated with the exogenous interaction effects.
The term WXρ captures the exogenous spatial interaction effects, where an independent variable (X) of one unit (e.g., unit A) influences the dependent variable (Y) of another unit (e.g., unit B). This term accounts for the spatial influence of explanatory variables across units.
#5 - Interaction Effect Among Error Terms (ρWu)
ρ represents a parameter associated with the interaction effect among error terms.
The term ρWu represents the interaction effect among the error terms of different units. In other words, it captures how the error term (u) of one unit is related to the error term of another unit, considering the spatial arrangement described by the matrix W.
This spatial econometric model is designed to account for the spatial dependencies and interactions that exist in the data. It allows for the consideration of endogenous and exogenous spatial effects, which can be particularly important in cases where observations in different units or locations are not independent but are influenced by their spatial proximity or relationships. Thus, the model parameters (ρ, θ, λ) are estimated to quantify the strength and nature of these spatial interactions, providing valuable insights for spatial data analysis.
Examples
Let us look at the spatial econometrics examples to understand the concept better-
Example #1
Let us create a hypothetical example to illustrate the concept of spatial dependence in the context of unemployment rates and labor force measures using spatial econometrics:
Scenario: Unemployment Rates In Counties
Suppose one wants to study the unemployment rates in a set of neighboring counties. They have collected data for several variables, including:
- Unemployment Rate (Y): The dependent variable is the unemployment rate in each county. This rate represents the percentage of the labor force in a county that is currently unemployed.
- Labor Force Participation (X1): An independent variable is the labor force participation rate, indicating the percentage of people in each county's population who are in the labor force.
- Educational Attainment (X2): Another independent variable is the average educational attainment in each county, measured as the percentage of the population with a college degree.
- Distance Between Countries (D): There is information on the physical distances between each pair of countries, reflecting how far they are from each other.
Now, let us consider the concept of spatial dependence:
Spatial Dependence Hypothesis:
One hypothesizes that the unemployment rates in neighboring counties are not independent of each other, as workers can move across county lines for employment opportunities. This spatial interaction between counties can lead to spatial dependence in unemployment rates.
Spatial Econometric Model:
A spatial econometric model is constructed to account for this spatial dependence:
Y = β1 * X1 + β2 * X2 + ρ * WY + ε
- Y: Unemployment rate in each county
- X1: Labor force participation rate
- X2: Educational attainment
- ρ * WY: The spatial interaction effect, where ρ is a parameter quantifying the strength of the spatial dependence, and W is a spatial weights matrix reflecting the spatial relationships between counties.
- ε: The error term representing unexplained variation.
In this model, ρ captures the degree of spatial dependence in unemployment rates. The spatial weights matrix W specifies the relationship between countries. The relationship can possibly be based on their geographical proximity or other criteria.
By estimating the parameters (β1, β2, ρ) and examining the spatial autocorrelation in the residuals (ε), one can assess whether the unemployment rates in neighboring counties exhibit spatial dependence. If spatial dependence is present, this model allows us to quantify its impact and incorporate it into our analysis. This leads to more accurate and informative results in the context of regional labor markets and policy decisions.
Example #2
In a study of housing prices within a city, the researcher posits that house prices are not solely determined by their intrinsic characteristics, such as size and distance to the city center, but may also be influenced by their proximity to neighboring houses. This hypothesis suggests that houses situated in close proximity to one another may exhibit similar prices due to shared neighborhood attributes.
Spatial Econometrics Model:
The researcher formulates a spatial econometric model to account for this potential spatial influence:
- Y: Represents the housing price
- X1: This signifies the size of the house.
- X2: Reflects the distance of the house to the city center.
- X3: Indicates the proximity of the house to public transportation.
- ρ * WY: Captures the spatial interaction effect, with ρ representing the degree of spatial influence and W detailing the spatial relationships among houses based on their spatial closeness
- ε: Represents the error term
This model enables the researcher to quantify the impact of spatial relationships on housing prices, thereby enhancing the comprehensiveness of the analysis and contributing to a deeper understanding of the dynamics within the city's housing market.
Applications
Spatial econometrics has a wide range of applications in various fields, as it helps researchers and policymakers better understand the impact of spatial relationships on economic, social, and environmental phenomena. Some common applications of spatial econometrics include:
- Regional Economics and Development: Spatial econometrics finds extensive use in analyzing regional economic disparities, regional growth, and the effectiveness of regional development policies. Researchers can assess how economic activities in one region affect neighboring regions and make informed decisions about resource allocation and development strategies.
- Urban and Real Estate Economics: One can employ spatial econometrics to examine housing markets, property values, and land use patterns in urban areas. It helps in understanding how proximity to amenities, transportation, and other urban factors impact real estate prices.
- Transportation Planning: Spatial econometrics is used to model transportation networks and their impact on accessibility, congestion, and travel behavior. This information is valuable for optimizing transportation infrastructure and traffic management.
- Health Economics: Researchers use spatial econometrics to study healthcare access, disease patterns, and the impact of healthcare policies. This is especially relevant in addressing healthcare disparities across regions.
- Agricultural Economics: One can apply spatial econometrics in agriculture to analyze crop yields, land use, and the spatial distribution of agricultural practices. Farmers and policymakers can make informed decisions about crop management and agricultural policies.
- Trade and International Economics: Spatial econometrics helps in examining international trade patterns and the determinants of trade flows. It helps in understanding how distance, transportation costs, and trade policies affect international trade.
- Social Sciences: Social scientists apply spatial econometrics to study various social phenomena, including education, labor markets, and social networks. It can provide insights into educational disparities, employment patterns, and the diffusion of social behaviors.