Table Of Contents
What Is Geostatistics?
Geostatistics is a specialized field of applied statistics that facilitates the prediction of spatial or temporal patterns through spatial modeling and interpolation. The three critical tools include semi-variograms, kriging interpolation, and standard error. Moreover, it even ensures measures of uncertainties by generating specific probable values for every location.
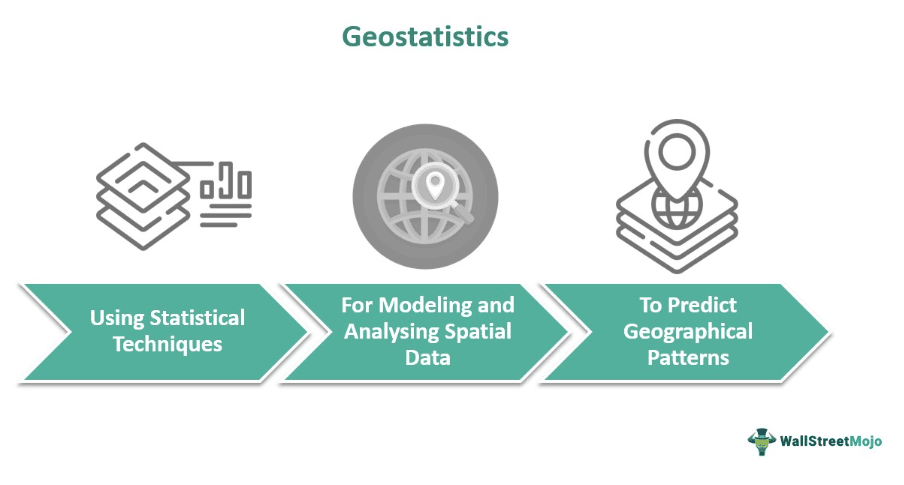
It integrates principles from statistics, mathematics, geology, and engineering for predicting values at unobserved locations. That is based on the available temporal data, making it a crucial tool in spatial analysis and decision-making processes. Moreover, geostatistical techniques are widely employed in geology, hydrology, environmental science, natural resource management, agriculture, urban planning, insurance and finance.
Key Takeaways
- Geostatistics refers to an applied statistics discipline that models and analyzes spatial or spatiotemporal datasets. It involves considering the geographical locations of data points and studying their patterns and correlations to make predictions.
- These methods are helpful when dealing with irregularly spaced or limited data points in geographical outreach.
- Furthermore, the three primary tools used in this field are semi-variogram, kriging interpolation, and standard error methods.
- Thus, geostatistics is used to analyze geographical data and make judgments in a variety of fields, including mining, geology, environmental science, hydrology, agriculture, natural resource management.
Geostatistics Explained
Geostatistics is a branch of statistics that deals with the analysis and interpretation of spatial data. Hence, this branch of statistics originated in the early 1960s through the pioneering work of the French mathematician Georges Matheron. Advances in computer technology facilitated the implementation of geostatistical techniques, leading to their widespread adoption in various scientific disciplines.
Moreover, the advancements extended to spatial modeling, interpolation methods, and various software tools. Today, it is an integral part of geology, petroleum engineering, environmental monitoring, agriculture, and urban planning. Thus, analyzing spatial patterns and making predictions based on such data is critical.
The following geostatistics analysis tools aid in understanding and utilizing spatial data, enabling informed decision-making across various fields:
- Semi-variograms: This tool draws all the possible data pairs as per the distance. On reaching a specific distance, the points are no longer correlated. An essential concept in geostatistics is spatial autocorrelation, which explores the correlation between data points at different locations.
- Kriging Interpolation: Krigging is used to create predictive models for spatial data. That establishes correlations between the temporal samples employing the semi-variogram mathematical function. Hence, its various kinds include co-krigging, universal, probability, indicator, and Empirical Bayesian kriging.
- Standard Error: This technique uses a standard error surface map that reflects the measure of confidence for the likeliness of acquiring the desired outcome. Therefore, it facilitates the estimation of uncertainty in the unsampled data.
However, the geostatistics modeling spatial uncertainty has several limitations. One crucial assumption is stationarity, assuming consistent statistical properties across the study area, which may not be accurate in real-world situations. Additionally, dealing with complex models and computational intensity, especially with large data sets, poses challenges. Furthermore, understanding and addressing geostatistics conditional bias is crucial for improving the accuracy and reliability of geostatistical predictions.
Principles
The following principles of geostatistics are crucial for applying its methods effectively and ensuring the accuracy of spatial predictions:
- Spatial Autocorrelation: Geostatistics interprets the spatial dependence in data, where nearby locations tend to have similar values.
- Variogram: It functions on this tool that quantifies spatial variability by measuring how the variance between data points changes with distance and direction.
- Kriging: It undertakes this geostatistical interpolation method to derive optimal estimates of unknown values at unsampled locations, incorporating spatial correlation information from the variogram.
- Anisotropy: This technique considers anisotropy when spatial correlation structure varies in different directions; using anisotropic variograms can provide accurate results.
- Stationarity: These methods assume stationarity where the statistical properties like mean and variance do not change in the long run.
- Measure of Uncertainty: It provides methods to quantify prediction uncertainty for making informed decisions.
- Cross-validation: Such models need validation through techniques like cross-validation to evaluate their predictive accuracy with new, unseen data points.
Examples
Let us understand the concept better with the help of examples.
Example #1
Suppose a chemical spill occurs at an industrial site. Environmental scientists can collect groundwater samples from various locations around the affected area. Geostatistical methods, such as kriging, can then be utilized to analyze these samples and create a contamination map. This map not only identifies the areas where contamination is present but also provides estimates of contamination levels in nearby unsampled locations.
Thus, environmental researchers and policymakers can install remedial systems, monitor the contaminant's spread over time, and assess the overall risk posed to human health and the environment.
Example #2
A report presents a groundbreaking approach to modeling interest rates by combining geostatistical methods and kriging forecasting techniques. Unlike traditional econometric strategies, the study uses a three-dimensional space to represent interest rates, rate maturity, and reference time. Specifically, it focuses on Euro Zero Rates (EZR), derived from the Euro interest rate swap market, which are essential in the European financial context. The research demonstrates the application of geostatistical techniques to accurately forecast interest rates, providing a new perspective on modeling the term structure of interest rates.
This paper introduces an innovative method for short-term interest rate forecasting, employing spatial statistical analysis of Euro Zero Rates' time series. Thus, the study develops an empirical anisotropic variogram and selects a theoretical variogram model for forecasting up to 12 months using Ordinary Kriging. Results from 2003–2014 indicate accurate long-term predictions, outperforming market proxies and occasionally surpassing the dynamic Nelson-Siegel model. Future research could expand comparative analysis, test longer-term predictions, and apply the method to other yield curves and historical periods.
Applications
Geostatistics analysis is a statistical discipline that deals with spatiotemporal datasets. It is a versatile concept that has various applications in different fields, including:
- Mining and Geology: Geostatistics in mining holds significance in estimating mineral reserves, predicting ore grades, and optimizing drilling locations.
- Disaster Management: These methods are employed in assessing risks pertaining to natural disasters like earthquakes, landslides, and floods for developing disaster management and risk mitigation strategies.
- Environmental Science: It helps in environmental monitoring, pollution assessment, and analyzing spatial patterns of environmental variables such as air and temperature.
- Natural Resource Exploration: Such techniques are applied for reservoir characterization, predicting oil reserves, and optimizing drilling locations in the oil and gas industry.
- Agriculture: Geostatistics facilitates soil analysis, crop yield forecast, and precision agriculture by gauging spatial variability of soil properties.
- Hydrology: It assists in groundwater modeling, rainfall prediction, and flood risk assessment by analyzing spatial patterns of hydrological variables.
- Epidemiology and Public Health: These techniques are employed to study the spatial distribution of diseases, analyze healthcare access, and predict disease outbreaks in different locations.
- Insurance and Finance: It is utilized in the insurance industry to assess and manage risks related to natural disasters or disease spread to decide appropriate premiums and manage their portfolios effectively.
- Urban Planning: It even helps in land use planning, transportation modeling, and analyzing urban growth patterns for sustainable urban development.