Although Bayesian and classical statistics have a similar application, they have distinct features. Let us look at their differences:
Table Of Contents
Bayesian Statistics Definition
Bayesian statistics in mathematics refers to a statistical tool used to validate uncertain events through conditional probability. The main purpose of this tool is to incorporate prior or new parameters into an event, making it easier to ascertain the probability of an uncertain outcome.
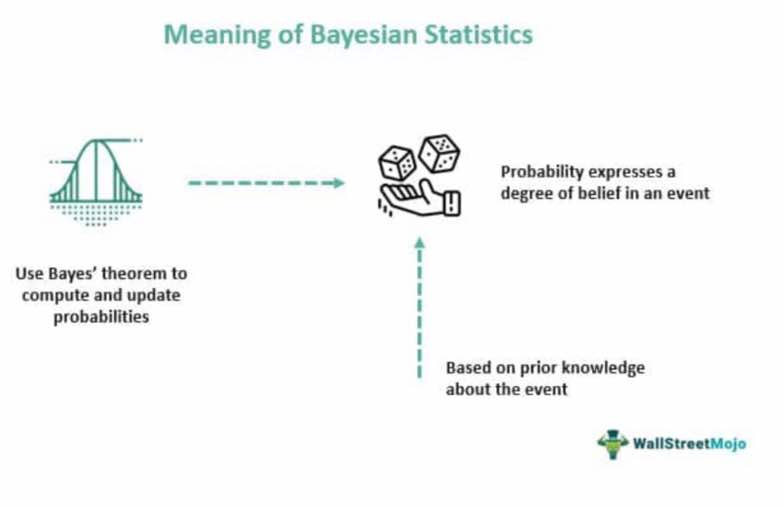
When dealing with two events (A and B), the initial step is to define the probability of an outcome. After framing the problem or hypothesis, it's essential to establish a prior belief by considering similar studies and experiments. Subsequently, data is collected, and Bayes's theorem is applied to the parameters, simplifying the determination of outcomes, especially for beginners.
Key Takeaways
- Bayesian statistics is a research tool utilized to ascertain the probability of events under uncertain conditions, employing the method of conditional probabilities.
- British statistician Thomas Bayes introduced this concept during the 18th century, presenting a paper to the Royal Society in 1763.
- The formula of Bayes theorem incorporates prior probability, likelihood, and posterior probabilities, proving effective for small and random sample sizes.
- This statistical model finds application in various fields, including machine learning, medical research, physics, finance, commerce, etc.
Bayesian Statistics Explained
Bayesian statistics is a statistical method that applies conditional probability to determine the occurrence of an event, taking into account previous outcomes with similar occurrences. The introduction of Bayesian statistics dates back to the 18th century in the United Kingdom. Pioneer and statistician Thomas Bayes presented his arguments in a paper to the Royal Society in 1763. However, the modern prevalence of the Bayesian concept emerged in the 1950s and 1960s.
The Bayesian statistics book uses various parameters and conditions, with probability being the major tool. It includes prior and posterior probability. Here, the prior probability considers events with similar occurrences, while the latter is the updated probability after observing a new data set. The combined effect of both results in Bayesian events.
Additionally, Bayesian statistics considers the likelihood of an event, aiding in determining how likely a hypothesis or event is to occur. This function observes a dataset, relates it to a hypothesis, and estimates the probability. Once estimated, users can apply the formula for Bayes's rule or conditional probability, as suggested in the Bayesian statistics book. The equation for Bayes's theorem is as follows:
P (A|B) = P (A â‹‚ B)/ P (B) = {P (A) * P(B|A)}/P (B)
Here, P (A) and P (B) are the respective events with their probabilities. P(A|B) depicts the probability of event A with B. The same goes for P(B|A), which depicts the probability of B with A. And lastly, P(A â‹‚ B) is the probability of both events occurring simultaneously.
Examples
Let us look at some examples to comprehend Bayesian statistics:
Example #1
Suppose Jenny is a researcher who wants to gather statistical data for her upcoming project. She wants to know the probability of average Americans in San Francisco consuming vegan food. So, she agrees to collect the data from and around a majority population. Here is the following data from 200 participants:
18-29 | 30-49 | 49-69 | 70 & more | Total | |
People consuming vegan food | 50 | 26 | 12 | 6 | 94 |
Not consuming vegan food | 30 | 40 | 20 | 4 | 94 |
Total | 80 | 66 | 32 | 10 | 188 |
In the above data, most participants consume vegan food in the teenage category. However, a similar total is visible in the other group, too. Also, Jenny wants to know the probability of the vegan category in the age group 30-49. So, let us apply the Bayes theorem in this case:
P (People consuming vegan food| Age group 30-49) = P (A â‹‚ B) / P (B)
= 26/66
= 0.39 or 39%
Here, the probability of participants consuming vegan food between the ages of 30 to 49 is 39%. It means, under the condition of age category, the event A (vegan food) turned conditional.
Here, the probability of participants consuming vegan food between the ages of 30 to 49 is 39%. It means, under the condition of age category, the event A (vegan food) turned conditional.
Example #2
In a recent study published by BMC Medical Research on October 26, 2023, Bayesian statistics demonstrated notable success in predicting patient treatment benefits in medical applications. For instance, researchers found these models to be effective tools when applying Bayesian parametric survival models to analyze population-level evidence from random clinical trials. Unlike other approaches, these Bayesian models require no hyperparameters and exhibit reduced susceptibility to overfitting issues, making them valuable for accurate and reliable predictions in medical contexts.
Applications
Since its inception, the introduction of Bayesian statistics has found widespread utility across various fields. Key applications include:
- Medical Science: Bayesian statistics plays a crucial role in medical research, aiding clinical researchers and doctors in determining the probability of a drug's effectiveness under uncertain conditions. It proves valuable in clinical trials during medicine development, contributing to preventing and managing disease spread by incorporating prior experiences.
- Finance and Commerce: The Bayesian model is instrumental in portfolio optimization and risk-return estimation in finance and business. Analysts leverage it to predict future estimates and rates, while creditors utilize the model to assess lending risks. For example, applying Bayes' tool allows firms to compare prior occurrences of debt for informed estimations of current repayment probabilities.
- Psychology: Applying Bayesian statistics in psychology facilitates understanding human behavior in various situations. It aids decision-making processes and helps acquire perceptions or opinions for specific policies.
- Bayesian Statistics in Machine Learning: In machine learning, Bayesian statistics finds significant implications in statistical inference, modeling, and experimental design. It assists in modeling uncertainty, particularly by incorporating neural networks into deep learning models.
- Astronomy and Physics: Scientists employ Bayesian statistics in astrophysics and related experiments to develop hypotheses and estimate their probabilities. This application enhances the scientific understanding of various phenomena.
- Environmental Science: Bayesian statistics, specifically the Bayes rule, can be applied in climate and weather-based models. This application makes it easier to calculate the probability of specific weather conditions in specific regions, contributing to advancements in environmental science.
Advantages And Disadvantages
Following are the benefits and limitations of Bayesian statistics in the research field. Let us look at them:
Advantages
- Bayesian statistics allows the incorporation of prior knowledge in the estimation process.
- The Bayes model provides a clear and intuitive understanding of uncertainty in statistical analysis.
- This statistical tool is particularly useful when working with small sample sizes.
- Unlike other statistical models, Bayesian statistics allows for data updates, leading to improved and more accurate results.
Disadvantages
- The model is prone to subjectivity with the incorporation of previous data, potentially leading to biased results.
- Implementing Bayesian statistics can be challenging due to its complexity. Users must have a solid understanding of probability models to use them effectively.
Bayesian Statistics vs Classical Statistics
Basis | Bayesian Statistics | Classical statistics |
---|---|---|
1. Meaning | It refers to a statistical tool for estimating probability under uncertain conditions. | Classical (also frequentist) statistics calculates probabilities in the long run. |
2. State of Parameter | The parameter is considered random. | The treatment for parameters remains fixed. |
3. Prior Knowledge | It includes previous information in the analysis. | No prior data is included in classical statistics. |
4. Sample Size | Bayesian statistics accommodates small sample sizes effectively. | Classical statistics is more suitable with large sample sizes. |
5. Nature | It is subjective, reflecting the degree of belief in the outcomes. | It is objective, based on defined probabilities. |