Following are the differences between Hurst Exponent and Augmented Dickey-Fuller Test:
Table of Contents
What Is Hurst Exponent?
The Hurst exponent is a statistical approach that quantifies the persistence present in a time series. If the series indicates possession of long-term memory, past events might impact future events. It indicates the presence, strength, and likelihood of a trend, showcasing its potential for continued growth.
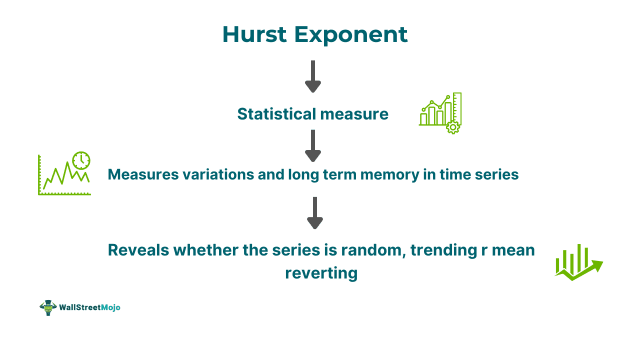
It is used to determine the underlying changes and predictability of a time series. The exponent is dependent on the time utilized for return calculation. It offers a quantitative framework to evaluate the degree of memory and predictability inherent in financial market data. The method aids data analysts in deciphering market dynamics and making informed decisions.
Key Takeaways
- The Hurst exponent is a measure used to determine the long-term memory of a time series.
- It quantifies the level of self-similarity and characterizes whether the series exhibits trending or mean-reverting behavior. Higher values indicate stronger persistence and the potential presence of trending patterns.
- It is derived from fractal dimension analysis and offers insights into chaotic time series data properties. The method helps reveal long memory processes and past patterns influencing future behaviors.
- The method estimates a dataset's recurring patterns, helping analysts determine if it's random white noise or if it reveals underlying trends.
Hurst Exponent In Trading Explained
The Hurst exponent is a singular scalar value that determines whether a time series is simply random, trending, or mean reverting. The variance present in a log price series is used to determine the rate of diffusive behavior. As a result, it can validate momentum or mean-reversion techniques. It serves as a crucial component for assessing the long-term memory of a time series, particularly in understanding the intricacies of the financial markets.
It aids in investigating the fractal nature of these markets. Moreover, it entails the tendency of market data to exhibit recurring patterns over various time scales. By estimating the Hurst exponent for a dataset, analysts can discern whether the data follows a purely random process of white noise or if it harbors underlying trends. This distinction is crucial as it elucidates the presence of autocorrelation, indicating the persistence of past values influencing future ones.
When autocorrelation exhibits a prolonged decay, it signifies a long memory process characterized by a Gaussian distribution. Surprisingly, seemingly random processes may exhibit Hurst exponent statistics indicative of long memory phenomena, a phenomenon termed colored noise.
Financial markets are complex amalgamations of deterministic chaotic and stochastic nonlinear systems. While they possess a weak deterministic component, they predominantly embody a strong inherent randomness. This strong randomness contributes to their chaotic nature. Moreover, financial markets operate as nonlinear feedback systems, where actions and reactions interact in a circular manner.
For instance, attempts at precise market prediction can significantly alter market conditions, underscoring the reflexivity inherent in financial systems. The method derived from fractal dimension analysis provides insights into the chaotic properties of time series data. It serves as a measure to determine the presence of long memory processes within the data, indicating whether past patterns persist and influence future behaviors.
Examples
Let us look at a few examples to understand the concept better.
Example #1
Imagine a trader, Dan, who utilizes the Hurst exponent to analyze a stock's price series. Dan finds it nearing 0.5, indicating a random walk behavior. This implies that the stock's price movements lack predictability and exhibit no clear patterns or trends. In such instances, short-term trading strategies reliant on pattern recognition may prove ineffective.
Traders should explore alternative approaches, such as long-term investment strategies or employing indicators and tools better suited to unpredictable market conditions. Comprehending this method offers valuable insights into price series behavior, enabling traders to adapt their strategies for enhanced market success.
Example #2
Introducing Hurst exponent in pair trading by J.P. Ramos-Requena, J.E. Trinidad-Segovia, M.A. Sánchez-Granero
In the paper, a novel methodology for pair trading is introduced, focusing on exploiting price movements of related assets. This new approach utilizes the Hurst exponent calculation, combining concepts of co-integration and mean reversion into a unified strategy. Results indicate that the Hurst approach outperforms classical Distance Method and Correlation strategies across various scenarios, demonstrating consistent performance and reduced drawdowns.
The proposed method employs the Hurst exponent to select pairs based on mean reversion and correlation principles. It showcased improved outcomes compared to traditional methods, especially with larger portfolios. Additionally, the model displays market portfolio returns neutrally and resilience during market downturns, making it a viable alternative or complement to existing pair trading strategies.
Significance
Some of the significant reasons for using the generalized Hurst exponent method are given as follows:
- Identification of trends: Utilizing the generalized Hurst exponent method enables the recognition of whether a financial market tends towards trending or mean-reverting patterns, aiding traders in formulating suitable trading approaches.
- Risk assessment and management: Understanding the method facilitates estimating potential price movement durations and magnitudes. It also aids in risk evaluation and determining optimal position sizes.
- Development of algorithmic trading strategies: Incorporating the method into algorithmic trading models helps capture signals indicative of trend-following or mean-reverting behavior, enhancing trading system performance.
- Analysis of market efficiency: Analysts can use the method to measure the degree of market efficiency. This is crucial for evaluating the validity of the efficient market hypothesis and making informed investment decisions.
- Portfolio optimization: The method contributes to portfolio construction by offering insights into the investment characteristics of various assets and their potential diversification benefits. This thus aids in the creation of well-balanced investment portfolios.
Hurst Exponent Vs. Augmented Dickey-Fuller Test
Basis | Hurst exponent | Augmented Dickey-Fuller test |
---|---|---|
1. Concept | It is a measure used to determine the long-term memory of a time series. | In statistical analysis, the augmented Dickey-Fuller test (ADF) examines the hypothesis that a time series sample contains a unit root. |
2. Essence | It quantifies the level of self-similarity or long-term dependence in a series, indicating whether it demonstrates trending or mean-reverting behavior. | The augmented Dickey-Fuller test (ADF test) is a widely used statistical test to assess the stationarity of a given time series. |
3. Aim | It offers valuable insights into the dynamics and predictability of a time series. | It aids in evaluating the suitability of modeling techniques and analyzing the characteristics of time series data. |