Table Of Contents
What Is Time Series Analysis?
Time series analysis refers to the method of analyzing a series of data points accumulated over a specific duration. It helps companies comprehend the underlying causes of systematic patterns or trends over time. Moreover, it can help organizations and individuals to clean their data.
With Time Series Analysis, one can detect patterns like trends, seasonality, and cycles over time
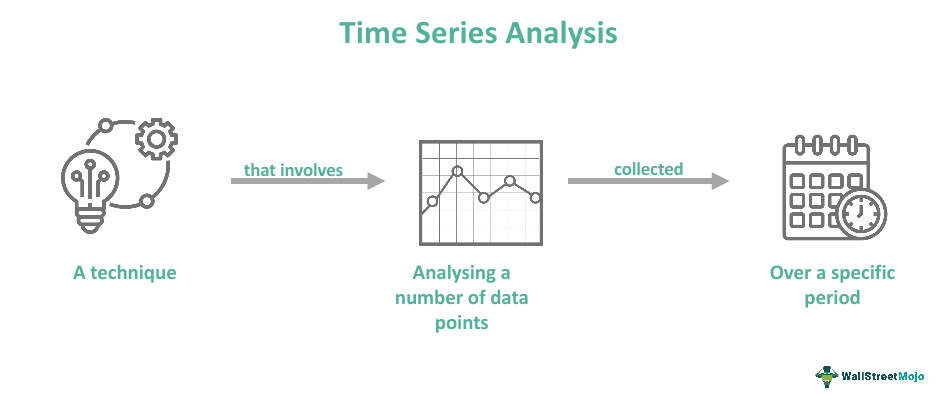
Typically, this type of analysis requires differentdata points to ensure reliability and consistency. This technique is common in different fields, for making predictions based on time, for example, stock market projections and weather forecasting models. There are different models of time series analysis. Some of them are intervention analysis, curve fitting, and descriptive analysis.
Key Takeaways
- Time series analysis refers to a technique used to analyze many data points collected during a particular timeframe to obtain useful statistics and characteristics. A key goal of this type of analysis is to project future value.
- Some models of time series analysis are descriptive analysis, classification, segmentation, and curve fitting.
- There are various advantages of time series analysis. For example, it enables organizations or individuals to clean their data. Moreover, it helps one comprehend the causes of trends over time.
- Such an analysis may not offer correct results in the long term.
Time Series Analysis Explained
Time series analysis in statistics refers to a technique that involves conducting an analysis of various data points collected during a certain timeframe. It provides insights and consequences of a given data set’s features changing over time. Individuals analyzing time series data record the data points at fixed intervals over a particular period. This type of analysis has applications in multiple areas, including economics, sales, and statistics. Let us look at a few reasons to consider conducting this type of analysis.
- Prediction: It can help predict stock prices. Traders or analysts can utilize this technique to know whether the price would increase or decrease and by how much.
- Features: One can conduct this kind of analysis to monitor features such as variability, seasonality, and trend.
- Inferences: It helps organizations and individuals to draw conclusions from data.
Since this kind of analysis shows the alteration of variables over time, it stands out from the rest. Time is an important variable, showing data adjustment throughout the final results and data points. It offers an extra source of information and a specific order of dependence between the data.
Components
The crucial components of this technique are as follows:
- Trend: Trend displays the usual tendency of data. It may go downward or upward over a specific, long period. The movement or direction may change over the duration. However, the trend’s tendency must remain the same.
- Cyclical Variations: These refer to the variations occurring in a time series that operate themselves for over a year. One entire period is a cycle. Generally, cyclical variations occur due to a combination of economic forces and their interactions.
- Random Or Irregular Movements: As the name suggests, these are irregular movements in a time series. Such fluctuations are unpredictable, uncontrollable, and erratic.
- Seasonal Variations: These variations are the alterations in a time series that occur in the short term. The variations are usually recorded as daily, hourly, weekly, monthly, and quarterly schedules. Seasonal variations take place owing to man-made conventions or natural forces. The different climatic conditions or seasons play a crucial role in such variations.
For example, the production of crops is heavily dependent on the seasons, and the sale of umbrellas rises during the rainy season.
Types
The different time series analysis models are as follows:
- Classification: It picks out and assigns categories or segments to the data.
- Descriptive Analysis: It involves identifying the patterns in the time series data along with uncovering trends, cycles, and seasonal variations.
- Explanatory Analysis: It explores the underlying factors that drive changes in the data and studies the impact of specific events or interventions on the time series data.
- Segmentation: It splits the data into different categories to disclose the source data’s underlying properties.
- Univariate Time Series Analysis: This method involves analyzing a single variable over time such as temperature, stocks, etc.
- Multivariate Time Series Analysis: It analyzes the relationship between two or more variables over time. We use it to analyze the impact of multiple variables on a given outcome.
To learn more of time series for analysis, you can check out our Time Series article here.
Examples
Let us look at this time series analysis example to understand the concept better.
This is the stock price chart of Colgate-Palmolive.
One can make the following observations by conducting a time series analysis.
- Trend: Between 2010 and February 2022, the stock was in an uptrend. This means that the price of the shares increased during that period.
- Random Movements: The chart shows random or irregular variations in the price owing to the dynamic economic environment.
Advantages And Disadvantages
The benefits and limitations of this technique are as follows:
Advantages
- It helps individuals and businesses analyze the historical data set and its patterns.
- This type of analysis creates the opportunity for persons to clean their data.
- It can help individuals gain a glimpse of the future, which can be quite useful.
- Time series data analysis can help one interpret the real meaning of the data.
- Another key advantage of time series analysis is that it helps understand the factors influencing specific variables in certain periods.
Disadvantages
- Time series data analysis does not support the missing values.
- The analysis may lead to inaccurate results in the long term.
- Data points must have a linear relationship for the technique to work.
- Since data transformations are compulsory, using this technique can be expensive.
- Human error could lead to the misidentification of the right data model. This can have a snowballing impact on the output.
Time Series Analysis vs Cross-Sectional Analysis
Those unfamiliar with the concepts of time series and cross-sectional analyses may think they are similar. We must know their distinct characteristics to understand how they differ. So. Let us look at the differences between the two concepts.
Time Series Analysis | Cross-Sectional Analysis |
---|---|
This technique considers the exact variables over a certain duration. | The cross-sectional analysis focuses on multiple variables at the same point in time. |
The profit earned by an organization over a duration of 3 years is an example of this method. | An example of this analysis is the maximum temperature recorded in different cities on a single day. |
Time Series Analysis vs Time Series Forecasting
Time Series Analysis
- It involves using different methods to analyze data and extract crucial characteristics and tactics related to it.
- Some of the methods of this analysis are the time domain method, parametric approach, and the frequency domain method.
- This refers to the technique individuals or companies utilize to analyze data points accumulated during a specific timeframe.
Time Series Forecasting
- Time Series Forecasting involves predicting future values according to the previously seen values utilizing the time series model.
- Some of the time series forecasting methods are the moving average, ARIMA (autoregressive Integrated Moving Average), and smooth-based models.
- This refers to the process of making scientific predictions on the basis of historical time-stamped data.