Table Of Contents
What Is Granger Causality Test?
Granger causality is a statistical hypothesis test used to determine whether one time series can be used to predict another. It identifies causal values between two variables and predicts time series.
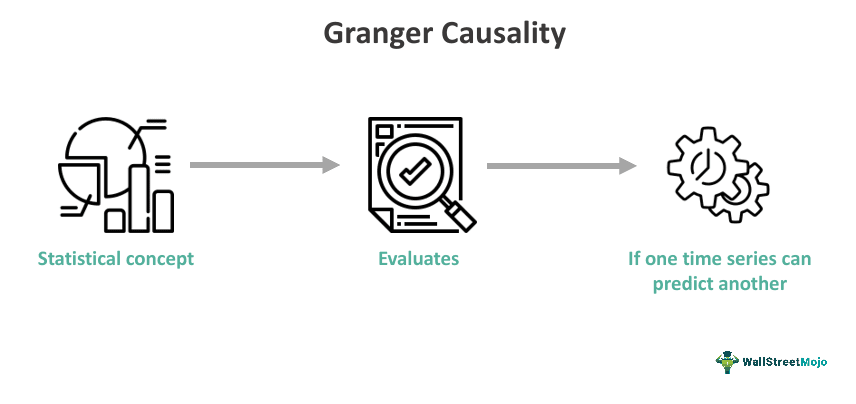
It helps in determining causal relations between economic variables like gross domestic product (GDP) and interest rates. Moreover, it also recognizes factors driving stock prices. Medically, it corroborates the relationship between various brain regions. Thus, it also predicts events like volcanic eruptions and earthquakes, while investors use it to understand the causal relationship between news sentiment and stock market volatility.
Key Takeaways
- Granger Causality is a statistical hypothesis test used to determine whether one time series can predict another.
- It involves defining a VAR model, conducting a granger-causality test, analyzing test results, and assessing causal impact.
- Moreover, it is easy to set up and use but only tests for predictive relationships, not true causation.
- These tests focus on temporal order, while Correlation quantifies linear association, and cointegration examines long-term equilibrium relationships between two series.
Granger Causality Test Explained
Granger Causality Test can be defined as a statistical hypothesis concept of causality to determine if one time series can successfully predict another time series. Econometric tests confirm whether a given variable is a good predictor of another. Additionally, to elucidate its functioning, researchers employ the vector autoregression (VAR) model. A VAR is a statistical approach that predicts a group of time series variables by using their past values and the past values of other variables linearly.
Furthermore, researchers conducted the Granger causality test by fitting a VAR model to different time series. They create two VAR models, with one being a reduced model incorporating delayed Y values. The other one is a full-scale model with delayed values of both X & Y. Therefore, the Granger causality test compares the performance of the two models of VAR. If the full VAR model outperforms the reduced model by a substantial margin, then one can conclude that X Granger causes Y.
It has many implications in different fields, including economics, finance, and neuroscience.
Therefore, the Granger causality approach is sensitive to the choice of time lags, and the results may vary depending on this choice. Hence, the Granger causality assumption states that there are no omitted variables that could be driving the observed relationships. It also assumes that the relationships between the variables should be linear.
In the financial world, it has led to many new trading strategies plus gaining insights into market dynamics. Policymakers & investors use it to examine the relationship between stock market returns and macroeconomic variables to make wise decisions regarding policies and investments. Additionally, researchers have applied it to risk management models to assess the relationships between various financial assets.
How To Interpret?
To understand Granger causality analysis, researchers examine the statistical significance of past values of one variable in predicting the future values of another variable. The following steps will help in interpreting it:
- Defining the VAR Model: To start, define a VAR model, including the relevant variables and their correlated values.
- Conducting a Granger Causality Test: Then, to find out whether one variable significantly influences another, use a Granger causality test. In this test, researchers compare the performance of an unconstrained model (including the predictor variable) with a restricted model (excluding it).
- Analyzing the Test Results: A test statistic, such as the Wald statistic or F-statistic, and the related p-value are produced by the test. Hence, the p-value denotes the association's statistical significance, whereas the test statistic quantifies the strength of the causal link.
- Assessing Causal Impact: If the p-value is less than a predefined significance level (e.g., 0.05), researchers demonstrate a substantial causal impact of the predictor variable on the target variable. Rejecting the null hypothesis indicates that the predictor variable is 'Granger-causal' concerning the dependent variable.
It's vital to remember that it only involves a statistical test that cannot establish causation.
Examples
Let us use a few examples to understand the topic.
Example #1
A company named Acme Corporation makes widgets. They wish to ascertain whether the sales of their AcmeWidget product and their advertising expenditures are causally related. For the previous two years, they have been gathering statistics on their AcmeWidget sales and advertising expenditures. Therefore, Acme Corporation uses the Granger causality test to examine the information.
The test's findings demonstrate a strong Granger causal link between AcmeWidget sales and advertising expenditure. It follows that an increase in advertising expenditure leads to a rise in AcmeWidget sales. Hence, with this information, Acme Corporation will be able to decide on its advertising budget with knowledge. For instance, they might spend more on advertising if they wanted to boost AcmeWidget sales.
Despite being purely hypothetical, this example shows how the Granger causality test may be applied to determine causal links between variables.
Example #2
Researchers offer a novel use of Granger causality in the financial industry in a recent news item titled "High-Dimensional Granger-causality Tests with an Application to VIX and News," which was published on August 4, 2023, in the Journal of Financial Econometrics. The study applies a new high-dimensional Granger-causality test to examine the link between news mood and the VIX index.
The authors find a strong causal association between news sentiment and the VIX index, suggesting that news sentiment may be used to anticipate future volatility in the stock market. Granger causality may be used to determine the causal linkages between variables in financial markets, as demonstrated by this particular discovery.
Additionally, the researchers use their results to create a novel trading technique that takes advantage of the causal relationship between news emotion and the VIX index. This study shows how Granger causality may be used in real-world situations and emphasizes how it can help guide investment choices in the dynamic financial world.
Advantages And Disadvantages
Advantages
- Very easy to setup, use, and implement
- Finds usage in establishing causal relationships between variables in multiple fields.
- These has multiple uses in trading strategies, identifying factors of market volatility, inflation and economic growth.
- Moreover, it can help in predicting natural calamities
- Helps in improving machine learning models
- They help in locating reasons for climate change and addressing them
- Furthermore, it can assist in improving public policy decisions and creating new scientific theories.
Disadvantages
- It does mean or imply true causation.
- Acts as a test for forecasting the relationship between variables
- Therefore, this test holds only time series data that remains stationary
- Relationships between cointegrated variables are not used with it.
- It isn't easy to interpret complex systems and requires comparatively long time series of data.
- Hence, it is not suited to every data type
- This concept may be unreliable in some cases.
Granger Causality vs Correlation vs Cointegration
All of them are useful in finding relationships between variables. However, discussed here are the major differences between the two:
Granger Causality | Correlation | Cointegration |
---|---|---|
The type of relationship between the variables involved is predictive. | Correlation is a type of relationship between the variables involved is linear. | Stationarity of the two-time series is required for the test |
Stationarity of the two- time series is required for the test | Here, stationarity is not required for the test | Stationarity is not required for the test |
Centers around the order of events. | Quantifies level of linear association | Based on long-term equilibrium relationship amongst two series |
Assesses causality using lagged values evaluation. | Shows only statistical relationships. | Addresses equilibrium and co-movement only. |
Uses F-statistics and p-values based on Granger-causality. | Deploys correlation coefficients like Pearson or Spearman. | An Augmented Dickey-Fuller (ADF) test is used. |
Indicates predictive causation but not true causation. | These indicate association or co-movement but not causation. | Implies a long-term relationship, suggesting common trends. |