Table Of Contents
What Is Backtesting Bias?
Backtesting bias refers to the potentially misleading results of backtesting a trading strategy. By evaluating the strategy on the same historical data used to fine-tune it, there is a risk of seeing better performance than what would likely be achieved in real-world trading conditions.
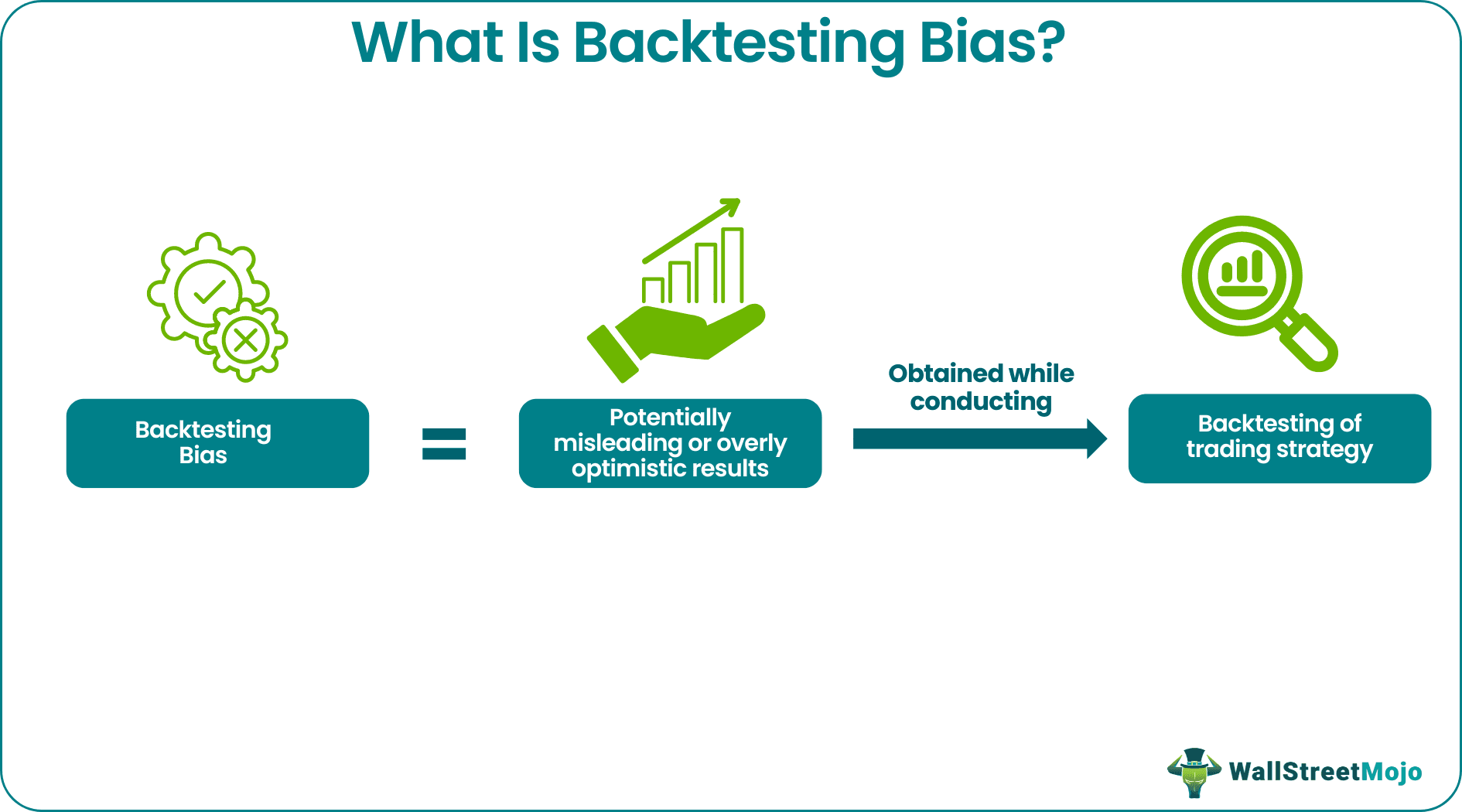
Backtesting bias can compromise the reliability of the outcomes and may influence investors to make incorrect decisions based on the backtesting results. By incorporating new and independent data, investors can safeguard against the pitfalls of relying solely on historical performance and make more informed judgments about the practical expectations of the strategy.
Key Takeaways
- Backtesting bias is the term used to describe the possibility of deceptive outcomes from backtesting a trading method.
- The goal of becoming aware of it is to assist investors, analysts, and others in making wise decisions following practical expectations, avoiding the traps of dependence on previous performance.
- To avoid backtesting bias, one can use separate historical data, select comprehensive datasets, and incorporate realistic assumptions.
- Survivorship bias, Cost of Carry/Holding Costs, inaccurate price simulation, and curve-fitting are known factors that can distort backtesting accuracy.
Backtesting Bias Explained
Backtesting bias refers to the potential distortion or misrepresentation of trading strategy results when using historical data for performance simulation. It can arise from limitations in data, unrealistic assumptions, and biases in the backtesting process. In backtesting, historical data is employed to evaluate a strategy's past performance. Still, bias can occur if the strategy is overly fitted to the data or fails to consider real-world constraints.
While backtesting offers valuable insights, it has limitations as it relies on historical data and assumptions that may not reflect future market conditions. Over-optimization and the inability to account for unforeseen events are common drawbacks. Backtesting bias is relevant across securities and assets, used by traders and analysts for strategy assessment.
Understanding and acknowledging backtesting bias is crucial for informed decision-making, considering real-world factors, and avoiding overreliance on historical performance.
Many traders use Saxo Bank International to research and invest in stocks across different markets. Its features like SAXO Stocks offer access to a wide range of global equities for investors.
Types
It is a fact that different types of biases can badly affect the accuracy and trustworthiness of backtesting in the financial industry. Therefore, one should know about the common types of backtesting biases:
- Black Swan Reconciliation: After black swan events, asset prices change due to significant price movements by brokers and exchanges, resulting in ambiguous backtest results.
- Survivorship Bias: It makes traders overlook bankrupt entities and failed processes and focus on successful ones. Thus, the trading strategies get skewed, and bias creeps in.
- Spreads: During volatile market conditions, the differences between buying and selling prices or bid & ask prices dynamically change. Thus, the accuracy of the backtesting gets hampered.
- Cost of Carry/Holding Costs: Backtest results get impacted if the interest rates or charges related to leveraged derivatives or positions fluctuate.
- Inaccurate Price Simulation: Using simulated price movements for high-frequency trading strategies by some backtesting platforms leads to skewed results.
- Change in Contract Specifications: When margin needs or settlement specifications have their product details modified by brokers or margins, the backtest outcomes get affected.
- Look-ahead Bias: Future market behavior knowledge used by brokers or exchanges while conducting backtesting leads to an unreal assessment of evaluations.
- Curve-Fitting and Optimization Bias: When past data is made to overfit a trading system, it leads to poor performance in future market conditions.
It is crucial to be aware of these biases to construct successful trading methods that can be adjusted to changing market circumstances and make educated investment decisions.
Examples
Let us use a few examples to understand the topic.
Example #1
Consider a scenario where Alex has traded EURUSD, initially valued at 1.1300. Suddenly, an unforeseen black swan event occurs, causing a substantial surge in EURUSD by 2000 pips, resulting in a price of 1.3300 (with one pip equal to $0.0001).
Considering this significant price movement, Alex enters a long position on EURUSD at 1.2300, corresponding to 1000 pips into the 2000 pips surge. As the price increases and reaches 1.3300, Alex seizes the opportunity to close his position, reaping a profit of 1000 pips.
However, an email informs Alex a few hours later that all trades will be settled at a price of 1.1800 due to the unexpected event. Consequently, Alex's initial profit of 2000 pips turns into a loss of 500 pips. This sudden reversal causes significant damage to Alex's account balance, resulting in substantial financial consequences. The example is inspired by a real-life incident involving Saxo trades consisting of a Swiss currency fiasco.
Example #2
A study on revisiting Gurufocus Predictability Rank 2010 examines the predictability of certain stocks using backtesting. The study focuses on the Gurufocus Predictability Rank but excludes dividend returns for predictable companies. This exclusion introduces a bias that favors the average returns of lower-ranked companies (one-star and two-star).
The backtesting study underestimates the average return for companies with a higher predictability rank by excluding dividend returns. This bias leads to misleading conclusions about the predictability and performance of stocks within the study.
This real-world example highlights how backtesting bias can occur when specific factors or data points are selectively excluded, skewing the results and potentially misrepresenting the true performance of investment strategies.
How To Avoid?
One must avoid backtesting bias to ensure a highly accurate assessment of trading strategies. It can be avoided in the following manner:
- Using unutilized historical data: By using a sample testing method to base the assessment on the left-out data set during strategy development validation, one avoids it as it helps check the strategy's performance in unknown market conditions.
- Ensuring robust data selection: The data set used for testing must be dynamic, comprehensive, and depictive of unforeseen market situations. Any cherry-picking of particular assets or periods could bias the outcome.
- Using logical presumptions: The backtesting process must implement realistic slippage, liquidity constraints, and transaction cost to consider real-world trading situations.
- Watching out for Look-Ahead Bias: One must not use any future data unavailable during the backtesting process. As a result, the trading strategy would not get biased performance.
- Using Walk-Forward Evaluation: Backtesting must also incorporate a new data set, assess, and make changes to the trading strategy periodically instead of entirely relying on historical data.
- Testing many strategies: One must apply the trading strategy to different assets and securities to test its efficiency and robustness throughout all market scenarios.
- Considering sensitization evaluation: the sensitivity of the backtesting process must be tested to know its performance across a range of parameter values.
- Ensuring unbiased validation: The backtesting process of trading strategy must seek expert opinion from external sources, professionals, or peers to verify the strategy's effectiveness and stand up to any challenge posed by possible flaws or biases.
- Assuring documentation and openness: All the records should be kept related to assumptions, methodology, any adjustments made, and data sources of the backtesting process. As a result, reproductivity and transparency would be apparent to the analysts.
Therefore, by simply putting these procedures into practice, traders and academics can reduce the chance of backtesting bias & acquire more trustworthy information on the effectiveness and feasibility of their trading techniques.
Disclosure: This article contains affiliate links. If you sign up through these links, we may earn a small commission at no extra cost to you.
Frequently Asked Questions (FAQs)
Backtesting bias can lead to false confidence in a trading strategy. If a strategy performs well on historical data but fails in live trading, it can result in significant financial losses. Backtesting is essential, but it can produce misleading results if not properly conducted, leading to poor investment decisions.
Several statistical techniques can be employed to detect potential backtesting bias, such as Monte Carlo simulations, walk-forward analysis, and sensitivity analysis. These techniques help assess the robustness of a strategy and identify any potential biases or flaws in the backtesting process.
While it is challenging to eliminate all forms of backtesting bias, one can take steps to minimize their impact. It includes using a diverse and representative dataset, incorporating transaction costs and slippage, avoiding data snooping practices, and conducting robustness tests on the strategy.