Nonlinear Regression
Last Updated :
21 Aug, 2024
Blog Author :
Edited by :
Raisa Ali
Reviewed by :
Dheeraj Vaidya, CFA, FRM
Table Of Contents
What is Nonlinear Regression
Nonlinear regression refers to a regression analysis where the regression model portrays a nonlinear relationship between a dependent variable and independent variables. In other words, the relationship between predictor and response variable follows a nonlinear pattern.
The nonlinear model is complex and, at the same time, creates accurate results. The analysis develops a curve depicting the relationship between variables based on the dataset provided. The model offering great flexibility can create a curve that best suits the scenario. This relationship can be anything from connecting time and population to investor sentiments and its nonlinear effect on stock market returns.
Table of contents
- Nonlinear regression refers to a regression analysis where the regression model portrays a nonlinear relationship between dependent and independent variables.
- It is more accurate and flexible than a linear model. The model can accommodate diverse curves deriving complex relations between two or more variables.
- Examples of this statistical model include the portrayal of the relationship between gold price & US CPI inflation and investor sentiments & stock market returns.
- Its application is seen in diverse disciplines like machine learning, insurance, forestry research, etc.
How Does Nonlinear Regression Work?
In nonlinear regression, the experimental data are mapped to a model, and mathematical function representing variables (dependent and independent) in a nonlinear relationship that is curvilinear is formed and optimized. It is accepted as a flexible form of regression analysis. Examples of algorithms used to develop nonlinear models are Levenberg-Marquardt nonlinear least squares and Gauss-Newton algorithms.
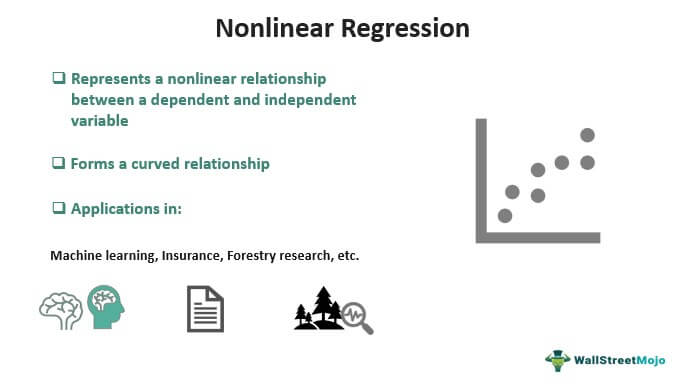
When the data shows a curvy relationship that is not a straight line applying a nonlinear model gives the accurate output. Unlike linear regression, there is no assumption of data linearity in the nonlinear model. In essence, when the data curve cannot be formed accurately using a linear method, the next option is the nonlinear method since it accommodates diverse types of curves. The fitness of the curve determines its correctness, and the curve illustrated reflects the curve's accountability to the data.
The nonlinear model can be parametric or non-parametric. The parametric model portrays dependent or response variables as a function of nonlinear parameters and independent or predictor variables. The model can also accommodate multiple response variables. Furthermore, machine learning methods are more suitable when it comes to non-parametric linear regression.
Example
Let's better understand the concept using the nonlinear relationship example between Gold and US CPI inflation. Gold investment is an effective hedge against inflation and currency depreciation in many countries. Hence analyzing the gold price movement is of great importance.
Even if the gold prices are stable to a great extent, they are affected by inflation, crude oil, etc. But the important one is the impact of inflation, and at the same time, gold prices can control the inflation instability. Therefore, a deep understanding of the relationship between inflation and gold price is a prerequisite.
In this case, nonlinear regression analysis is employed for analyzing data. The dependent variable is gold price, and the independent variable is inflation. The regression analysis results revealed that inflation impacts the gold price. The possible explanation why gold does not always move with CPI increases is that gold is considerably affected by large spikes in inflation. Still, small increases in inflation or dropping inflation have little impact on gold's price upward or downward trajectory.
Application
The nonlinear regression models are predominantly used for prediction, financial modeling, and forecasting purposes. The nonlinear model is used in many fields and sectors like insurance, agriculture, finance, investing, machine learning AI, and understanding broader markets. Let's look into some of the significant applications:
- Since most biological processes are nonlinear in nature, we can find nonlinear model applications in forestry research. A simple power function to relate tree volume or weight in relation to its diameter or height is an example.
- The use of a nonlinear model in developing a wide-range colorless gas, HCFC-22 formulation is an example from the field of Chemistry.
- In research and development, it is used in the process of formulation of the problem and deriving statistical solutions to the calibration problem.
- It is used in the insurance domain. For example, its usage can be seen in the computation of IBNR reserves.
- It is of great importance in agricultural research. Because many crops and soil processes are better captured by nonlinear than linear models.
Frequently Asked Questions (FAQs)
One of the formulas used to represent the nonlinear model is listed below.
Y = f(X,β) + ϵ.
Where f is the regression function and ϵ is the error term while X β are vector parameters.
Furthermore, performing nonlinear regression in excel is a famous method. It develops scatterplot and polynomial trendlines based on the given dataset.
The nonlinear model is more flexible and accurate. Although both models can accommodate curvature, the nonlinear model is significantly more versatile in terms of the forms of the curves it can accept.
It's a method for performing more flexible nonlinear analysis to obtain proper outputs such as choices, categorization, or inferences when similar future states or inputs are present. This method can provide impressive results and frequently beats people in performance, stability, and precision.
Recommended Articles
This has been a Guide to What is Nonlinear Regression. We explain the nonlinear regression analysis, model, examples, applications like in machine learning. You may also have a look at the following articles to learn more –