Table Of Contents
Difference Between Covariance Vs Correlation
Covariance Vs Correlation gives us the differences between the two statistical concepts used to determine the relationship between two random variables and that are exactly opposite to each other. However, they both are used in statistics and regression analysis. Covariance shows us how the two variables vary or differ from each other, whereas Correlation shows us the relationship and how they are related, i.e., how modification of one variable impacts the other.
For example, let us express these concepts mathematically for two random variables, A and B, with mean values as Ua and Ub and standard deviation as Sa and Sb, respectively.
Effectively we can define the relationship between the two:

Both Correlations and Covariance find application in statistical and financial analysis fields. Since Correlation standardizes the connection, it is helpful in the comparison of any two variables. In addition, it helps analysts develop strategies like pair trade and hedging for efficient returns on the portfolio and safeguarding these returns in terms of adverse movements in the stock market.
We will consider some real-time values to understand the differences or the relation between them with specific examples.
Table of contents
- Covariance and Correlation are very closely related to each other, and yet they differ a lot.
- Covariance defines the type of interaction, but Correlation represents the type and the strength of this relationship. Due to this reason, Correlation is often termed as the special case of Covariance.
- Most analysts prefer Correlation as it remains unaffected by the changes in dimensions, locations, and scale. Also, since it is limited to a range of -1 to +1, we can compare variables across domains. However, an important limitation is that these concepts measure only the linear relationship.
What Is Covariance?
Covariance measures how the two variables move concerning each other and is an extension of the concept of variance (which tells about how a single variable varies). It can take any value from -∞ to +∞.
- The higher this value, the more dependent the relationship is. A positive number signifies positive Covariance, that means that an increase or variation will correspondingly increase in the other variable, provided other conditions remain constant.
- A negative number signifies negative Covariance, which denotes an opposite relationship between the two variables. Though Covariance is perfect for defining the type of relationship, it is not good for interpreting its magnitude.
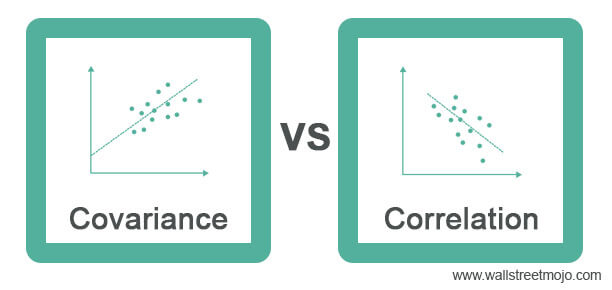
Covariance Formula And Example
Let us understand the covariance formula to calculate and assess the movements of variables. The formula is,

where,
- RAi =Return of stock A in the ith interval
- RBi =Return of stock B in the ith interval
- RA=Mean of the return of stock A
- RB=Mean of the return of stock B
- n = Sample size or the number of intervals
The covariance calculation between stock A and stock B can also be obtained by multiplying the standard deviation of returns of stock A, the standard deviation of returns of stock B, and the correlation between stock A’s and stock B’s returns.
Mathematically, it represents as,
Cov (RA, RB) = ρ(A, B) * ơA * ơB
where,
- ρ(A, B) = Correlation between returns of stock A and stock B
- ơA = Standard deviation of returns of stock A
- ơB = Standard deviation of returns of stock B.
Let us consider an example to understand the Covariance.
Consider the data given below of stock A and stock B with the following daily returns for three days.
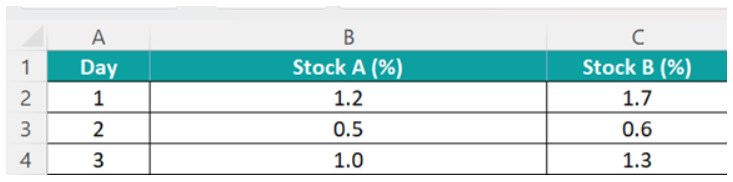
We will determine the covariance between stock A and stock B.
Given, RA1 = 1.2%, RA2 = 0.5%, RA3 = 1.0%
RB1= 1.7%, RB2 = 0.6%, RB3 = 1.3%
Therefore, the calculation will be as follows,
Now, mean return of stock A, RA= (RA1 + RA2 + RA3 ) / n
- RA= (1.2% + 0.5% + 1.0%) / 3
- RA= 0.9%
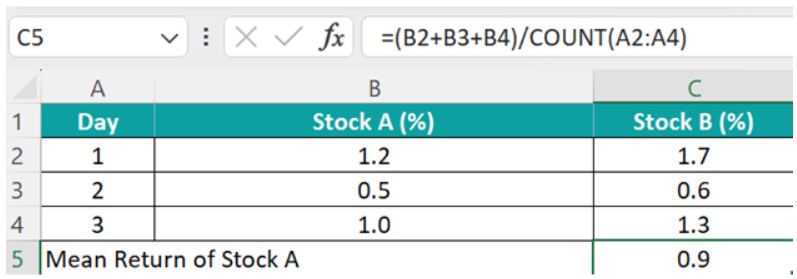
Next, Mean Return of Stock B, RB= (RB1 +RB2+ RB3 ) / n
- RB= (1.7% + 0.6% + 1.3%) / 3
- RB= 1.2%
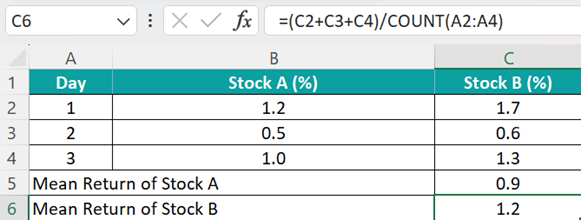
Therefore, one can calculate the covariance between stock A and stock B as:

Covariance between Stock A and Stock B will be –
=((B2-C5)*(C2-C6)+(B3-C5)*(C3-C6)+(B4-C5)*(C4-C6))/(COUNT(A2:A4)-1)
= / (3 -1)
= 0.200
- Therefore, the Cov(RA, RB) = 0.200
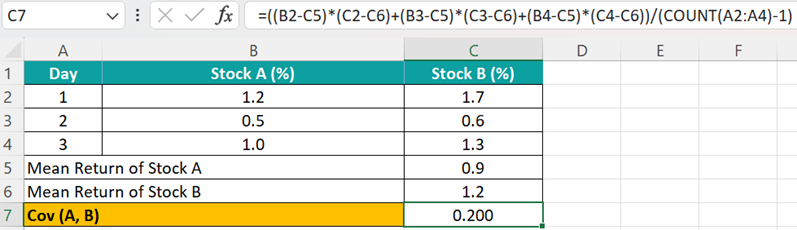
Therefore, the covariance between stock A and stock B is 0.200, as shown above, which is positive. As such, both returns move in the same direction, i.e., either with positive or negative returns.
What Is The Correlation?
Correlation is a step ahead of Covariance as it quantifies the relationship between two random variables. In simple terms, it is a unit measure of how these variables change concerning each other (normalized Covariance value).
- The Correlation has an upper and lower cap on a range, unlike Covariance. It can only take values between +1 and -1. A Correlation of +1 indicates that random variables have a direct and strong relationship.
- On the other hand, the Correlation of -1 indicates a strong inverse relationship, and an increase in one variable will lead to an equal and opposite decrease in the other variable. 0 means that the two numbers are independent.
Correlation Formula And Example
If we have two variables, x, and y, then the correlation coefficient between 2 variables can be found as:
Correlation Coefficient = ∑(x(i)- mean(x))*(y(i)-mean(y)) / √ (∑(x(i)-mean(x))2 * ∑(y(i)-mean(y))2)
where,
- x(i)= value of x in the sample
- Mean(x) = mean of all values of x
- y(i) = value of y in the sample
- Mean(y) = mean of all values of y
Let us consider an example to understand the Correlation.
Below are the values of x and y, with all the necessary details.
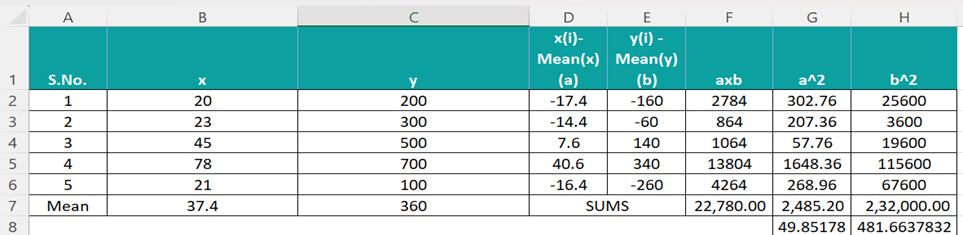
The calculation is as follows.
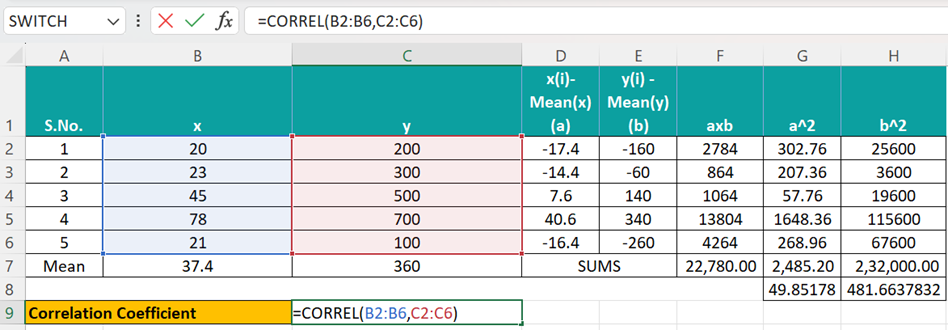
Basis Excel formula = CORREL (array(x), array(y))
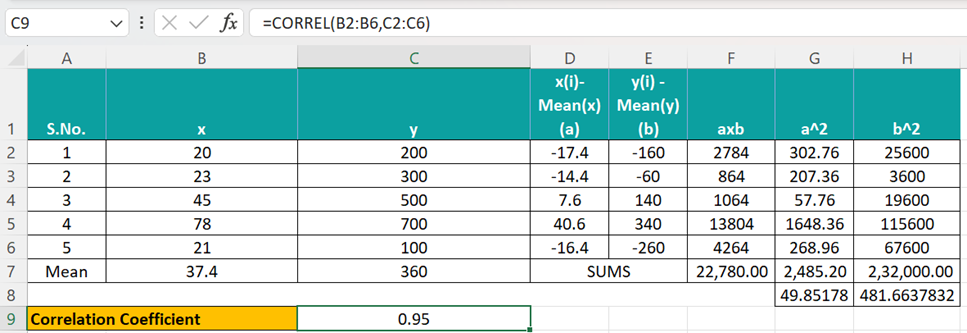
Therefore, the Coefficient = +0.95
Since this coefficient is near +1, x and y are highly positively correlated.
Correlation Vs Covariance Infographics
Let us see the top differences between Correlation and Covariance.
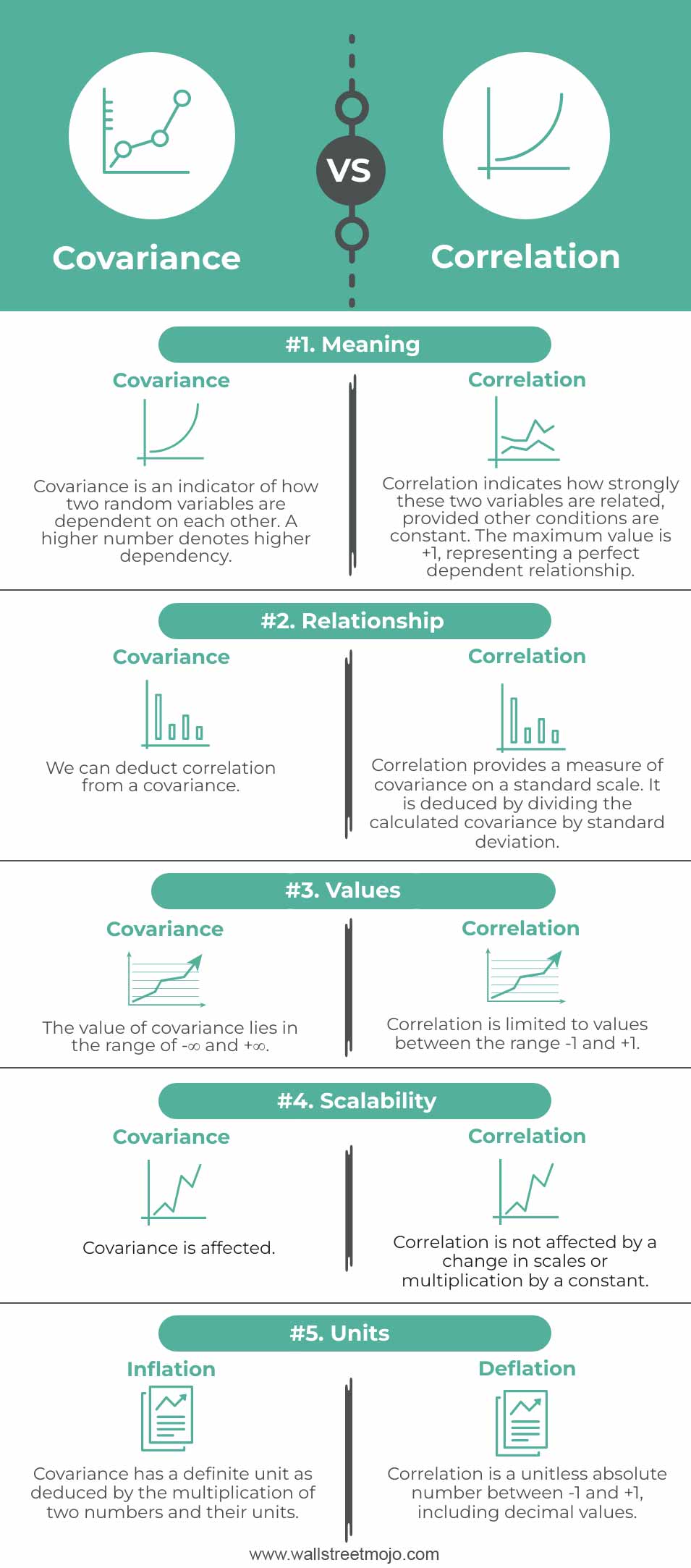
Correlation Vs Covariance Key Differences
- Covariance is an indicator of how two random variables change concerning each other. Correlation, on the other hand, measures the strength of this relationship. The correlation value is bound to the upper by +1 and the lower by -1. Thus, it is a definite range. However, the range of Covariance is indefinite. It can take any positive or negative value (theoretically, the range is -∞ to +∞). You can rest assured that a Correlation of .5 is greater than .3, and the first set of numbers (with a Correlation of .5) are more dependent on each other than the second set (with a Correlation of .3). Interpreting such a result would be tough from Covariance calculations.
- Change of scale affects Covariance. For example, if the value of two variables is multiplied by similar or different constants, this affects these two numbers’ calculated Covariance. However, applying the same mechanism for Correlation, multiplication by constants does not change the previous result. That is because a change in measurements does not affect Correlation.
- Correlation defines the inter-dependence of two variables that helps us to compare any two variables irrespective of their units and dimensions.
- We can calculate Covariance for only two variables. On the other hand, Correlation can be calculated for multiple sets of numbers. Another factor that makes the Correlation desirable to analysts compared to Covariance.
Covariance Vs Correlation Comparative Table
Basis | Covariance | Correlation |
---|---|---|
Meaning | Covariance indicates how two random variables are dependent on each other. A higher number denotes higher dependency. | Correlation indicates how strongly these two variables are related, provided other conditions are constant. The maximum value is +1, representing a perfect dependent relationship. |
Relationship | We can deduct Correlation from a Covariance. | Correlation provides a measure of Covariance on a standard scale. It is deduced by dividing the calculated Covariance by the standard deviation. |
Values | The value of Covariance lies in the range of -∞ and +∞. | Correlation is limited to values between the range -1 and +1. |
Scalability | Covariance is affected. | Correlation is not affected by a change in scales or multiplication by a constant. |
Units | Covariance has a definite unit as deduced by the multiplication of two numbers and their units. | Correlation is a unitless absolute number between -1 and +1, including decimal values. |
Recommended Articles
This article is a guide to Covariance vs Correlation. Here we learn relation & difference between Covariance & Correlation, examples & downloadable templates. You may also have a look at the following articles: -